Innovating Land Mapping and Accessibility for Sustainable Development
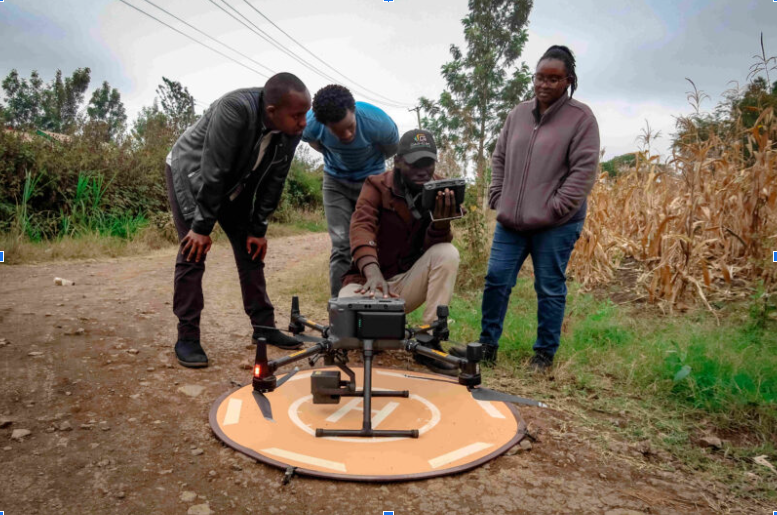
Spatial Collective, Radiant Earth, the Kenyan Space Agency (KSA), and PLACE collaborated on a pilot project to collect and curate labeling high-resolution agricultural data for open access and public benefit in Kenya. This post describes the practical steps taken by Spatial Collective during the project implementation, encompassing image, drone, and flight requirements, data collection, image processing, and image labeling.
Introduction and Background
In Sub-Saharan Africa, the challenge of documenting rural land persists. According to some estimates, 60 to 90% of rural land remains unrecorded. Historical constraints include the high costs associated with acquiring data, including obtaining high-resolution aerial imagery, and limited access to technology. The scale of the task – Africa is a vast continent – and the commercial sector’s hesitance to share data openly further compounded this challenge. However, in recent years, a shift has occurred as technological costs have decreased, allowing governments and even individuals to collect essential data.
In response to this pressing need for accessible land imagery, our pilot project harnessed the capabilities of drones, advanced imaging sensors, artificial intelligence, and cloud-native data-sharing. Focused on Murang’a County, Kenya, our initiative aimed to capture high-resolution aerial imagery spanning both agricultural and urban land. The primary aim of the project encompassed not just capturing the imagery but also labeling it for the purpose of training machine learning models, automating the identification of field boundaries and building footprints.
Simultaneously, the project sought to democratize access to this imagery by making it widely available for all stakeholders. The collected and labeled imagery forms the basis for addressing governmental requirements for data, streamlining land surveys, guiding urban planning decisions, and enhancing agricultural policies. Furthermore, these resources are invaluable for analyzing aspects such as land use patterns, environmental impacts, socio-economic indicators, and resource sustainability, marking a significant step in filling critical gaps in land documentation and regional planning.
Step One: Image Requirements
Aerial imagery is the cornerstone of this project. Prior to conducting the data collection and labeling process for aerial imagery, it was important to understand the specific image requirements. Technical details included imagery in RGB format to ensure a natural color representation, images had to be georeferenced and have an average Ground Sample Distance (GSD) of 5cm or higher. Additionally, the images were required to be captured on clear days to minimize shadows and cloud cover, with a side overlap of 70% and a forward overlap of 80%. Ensuring a radiometric resolution of 24-bit (3 x 8 bits per band) was also essential to maintaining a palette of 16.7 million color values for accurate processing and analysis.
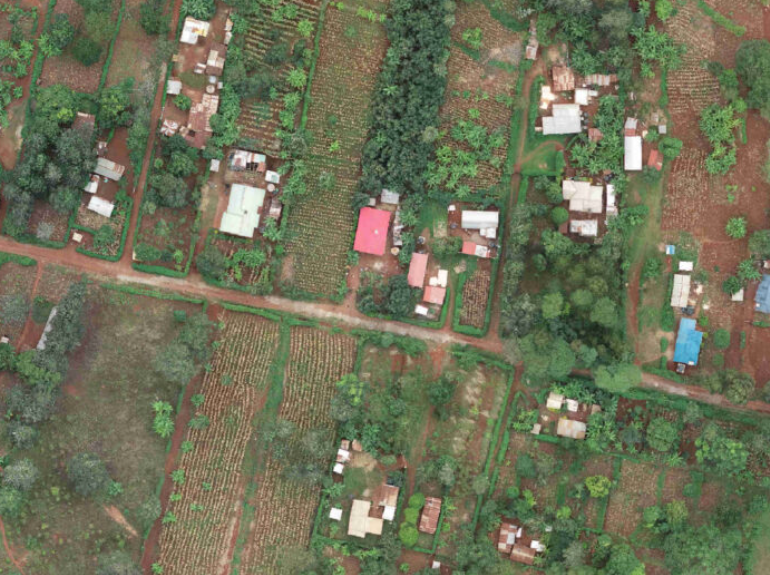
Step Two: Drone Selection
After understanding the specific image parameters, we had to select an appropriate Unmanned Aerial Vehicle or drone. To effectively capture the required imagery, the drone needed to have several key specifications. These included a fixed focal, wide-angle lens with a minimum focal length of at least 55% of the sensor diagonal or 67% of the sensor width. Additionally, the drone’s camera was required to have a pixel pitch ranging from 4 to 6 microns, with image stabilization turned off. It was crucial for the camera to record the date and time accurately to the nearest second on the local time system while also documenting each image’s filename together with other essential EXIF data for cataloging and analysis.
Following these specifications, the choice was the DJI Matrice 300 RTK drone with a Zenmuse L1 Sensor. This drone was locally accessible, and, moreover, the selected drone complied with registration by the Kenyan Civil Aviation Authority (KCAA) and maintained appropriate insurance coverage. Spatial Collective partnered with Geo-Cart Surveys and Engineering Solutions, a Kenyan company that provided the drone and supported the project with aerial mapping.
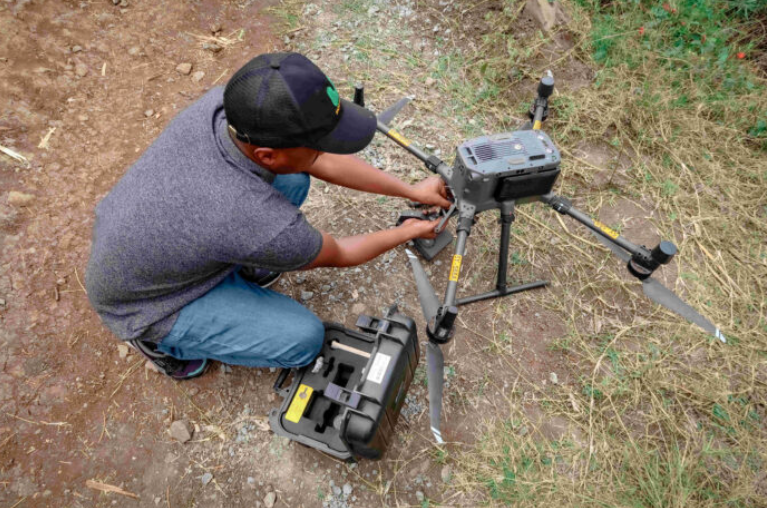
Step Three: Drone and Flight Operating Requirements
For the drone to be operational in Kenya, we ensured that the drone possessed a valid UAV Registration Certificate and Drone Insurance. Additionally, the operators were required to hold a Remote Operator Certificate, along with a Pilot and observers’ Remote Pilot License. To conduct drone flights effectively within the region, we secured specific permissions. This included obtaining a Letter of No Objection from the local authorities, particularly the Murang’a County Government, as well as an Authorisation Letter for Drone Survey. The application to obtain the authorization encompassed an official request for drone mapping, delineation of the Area of Interest for drone activities, submission of a detailed flight plan outlining the number of days necessary for drone operations, and providing the names of pilots alongside their Remote Pilot’s License numbers.
Step Four: Area Selection and Data Requirements
Our primary focus was to create 1,500 buildings and 3,000 agricultural labels within the Kenol area in Murang’a County, meeting the specific data criteria essential for subsequent machine learning applications. Kenol area was chosen due to its status as a swiftly expanding region encompassing both urban and rural land uses, making it an ideal location for this data collection initiative. Moreover, Kenol provided ease of access for obtaining flight approvals from the KCAA, ensuring adherence to safety and operational guidelines. To meet the data requirements, approximately 10 square kilometers were identified as sufficient within this area for data acquisition and label generation.
Step Five: Data Collection
The data collection process involved several stages, including the Flight and Safety Plan, Flight Setup, Data Collection and Monitoring, and Image Capture and Storage.
The Flight and Safety Plan encompassed the detailed design of a flight plan, considering the area’s terrain and desired outputs. This plan involved identifying launch points to optimize operational efficiency while ensuring a continuous visual line of sight of the drone. Additionally, local authorities, such as the area chief and police, were informed of the drone operations to prioritize public safety during the flights.
Flight Setup was carried out using a Ground Control Station (GCS) that precisely positioned the drone while in flight. The drone platform, overseen by a Remote Operator/Pilot, facilitated remote monitoring to ensure compliance with operational parameters. Pre-programmed, the drone executed its tasks autonomously, occasionally requiring minimal user inputs.
Data Collection and Monitoring involved the autonomous implementation of a pre-programmed flight plan by the drone, remotely supervised by the drone pilot through a radio link. The pilot and observers maintained a constant visual line of sight throughout the operation.
Image Capture and Storage centered on the use of the onboard Zenmuse L1 sensor to capture images, storing them on the drone’s SD card. The drone, following pre-programmed instructions, automatically captured images at set intervals according to specific image specifications. These images were then transferred to an external hard drive for backup and subsequent post-processing. The overall outcome included the capture of 8,096 images, covering an area of 11.32 square kilometers.
Step Six: Image Processing
PLACE managed the image processing and storage. This included a series of steps to transform individual images into a singular, comprehensive depiction of the area through a process known as ortho-rectification. Ortho-rectification involves the geometric correction of images to eliminate terrain effects and distortions, resulting in orthophotos where measurements within the image accurately correspond to measurements on the ground. The post-processing phase required several procedures: uploading all individual images to a cloud server via File Transfer Protocol, establishing a cloud-based image processing server instance, and installing Agisoft Metashape Pro, a crucial photogrammetry pipeline tool. Using Agisoft Metashape Pro, the process involved calibrating images in accordance with the specifications of the Zenmuse L1 camera, aligning photos through Aerial Triangulation based on their respective capture locations, employing orthorectification algorithms to generate meshes and Digital Elevation Models, and producing a georeferenced orthophoto in Tiff format, which was made available for transfer and further use.
Step Seven: Labeling
Once the imagery was processed, the subsequent phase involved the final step of labeling the imagery. Labeling in the context of machine learning relates to the process of associating particular objects or features within the data, such as images, with relevant annotations. To facilitate this process, we utilized the Kili Platform. Two dedicated labeling teams were established: one composed of interns from the Kenya Space Agency (KSA) and other KSA stakeholders tasked as labelers, while the second comprised staff from KSA and Spatial Collective, serving as administrators responsible for ensuring and maintaining quality standards.
Ultimately, a total of 12,651 annotations were done for both buildings and fields. These annotations are instrumental in further training machine learning models, providing them with the necessary data to recognize and interpret various elements within the imagery.
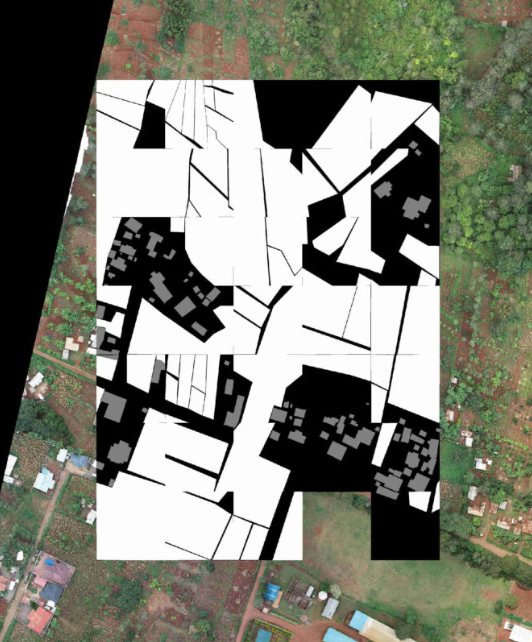
Step Eight: Workshop
The final phase involved a technical workshop prepared and carried out by Spatial Collective and the Kenya Space Agency. This workshop included active participation from a variety of stakeholders, including representatives from the Kenyan and Murang’a governments, civil society organizations, universities, and the private sector.
—
For more information on the project, get in touch with Charles Mwangi at the Kenyan Space Agency or Primoz Kovacic at Spatial Collective.